
Headlines are crafted to grab attention and that’s exactly what this New York Times article did:
"Nevada Asked A.I. Which Students Need Help. The Answer Caused an Outcry."
"The new system cut the number of students deemed “at risk” in the state by 200,000, leading to tough moral and ethical questions over which children deserve extra assistance." - New York Times
That might be as far as many people read before becoming morally outraged and hitting the share button. However, I’ve been doing some work in the K-12 sector recently and I wanted to better understand the particulars, such as which AI system? Who built it? What data was used? How exactly did it arrive at this outcome? I was also curious as to what led Nevada to engage an AI vendor in the first place.
What I found was not a clear cut case of algorithmic bias, but a lesson about the ways that we misuse data, regardless of whether or not AI is involved.
Free lunch = At-risk
In Spring 2023, two researchers published an article, based on their work, which called into question the validity of using program enrollment data for ‘free lunches’, formally called the National School Lunch program, as a means to identify children in need. For years, this data has been used as a proxy metric for students experiencing poverty and thus, deemed to be at risk. The data has been relied on as a means to distribute funding to school districts. However, the research suggests that for a number of reasons, the data is inflated and does not accurately represent in need children. The researchers called this data ‘grossly inaccurate’. To summarize the main points:
Community Eligibility Provision - if a school or district is deemed ‘sufficiently high poverty’ - every student in the school or district gets enrolled in the program.
Misaligned incentives - school districts with higher numbers will get more funding, parents can get a free lunch to offset costs, school staff want to err on the side of caution to ensure nutritional needs are met
Lack of enforcing the rules - The US Department of agriculture who is tasked with oversight was not particularly interested in enforcing the rules and there were few consequences for misrepresenting the data
The problem isn’t necessarily handing out free lunches to students. Rather, the core issue is when data used for one thing is assumed to be a good proxy for another.
Free lunch data is not the same thing as family income - which is a better, but harder to access, indicator of need.
The researchers used two other sources of poverty data based on income - direct certification, which indicates a family's enrollment in a social welfare program, and neighbourhood poverty. If the lunch enrollment data was accurate it should align with these other sources, but the researchers found it was up to 40% misaligned, or over-subscribed. The impacts of this data are enormous because of how it is used.
“the federal Every Student Succeeds Act requires states to track gaps in student achievement by poverty status. Among the 50 states, 44 use free and reduced-price lunch enrollment to identify low-income students. These data are also commonly used to allocate federal, state, and local funding to schools serving low-income children. School and district poverty rates, as determined by free and reduced-price lunch enrollment, additionally feature prominently in social science research, school-funding lawsuits, state laws and regulations, and philanthropic investment.” - Education Next
I wondered if this research itself had any other hidden agendas - such as being funded by interests with an ideology to defund education. I didn’t find any evidence of that being the case and the work has been published in credible sources. While the research was conducted in one state, Missouri, the researchers also conducted the same work using a 27 state sample with similar findings, suggesting this is a wider spread issue.
Nevada gets a failing grade
One of the first questions I ask in doing an assessment of an AI system is why AI? What’s driving the decision for an organization to change whatever methods they are using to solve a problem and seek an AI/ML (artificial intelligence/machine learning) solution?
The New York Times report alludes to Nevada’s school funding being ‘lopsided’ and ‘wanting to improve its status’. Nevada’s decision to embrace AI/ML may have been partially driven by public embarrassment and a desire to try and do better. In a report called Making the Grade, Nevada was given a failing score across the board when it comes to three areas - funding level, funding distribution and funding effort.
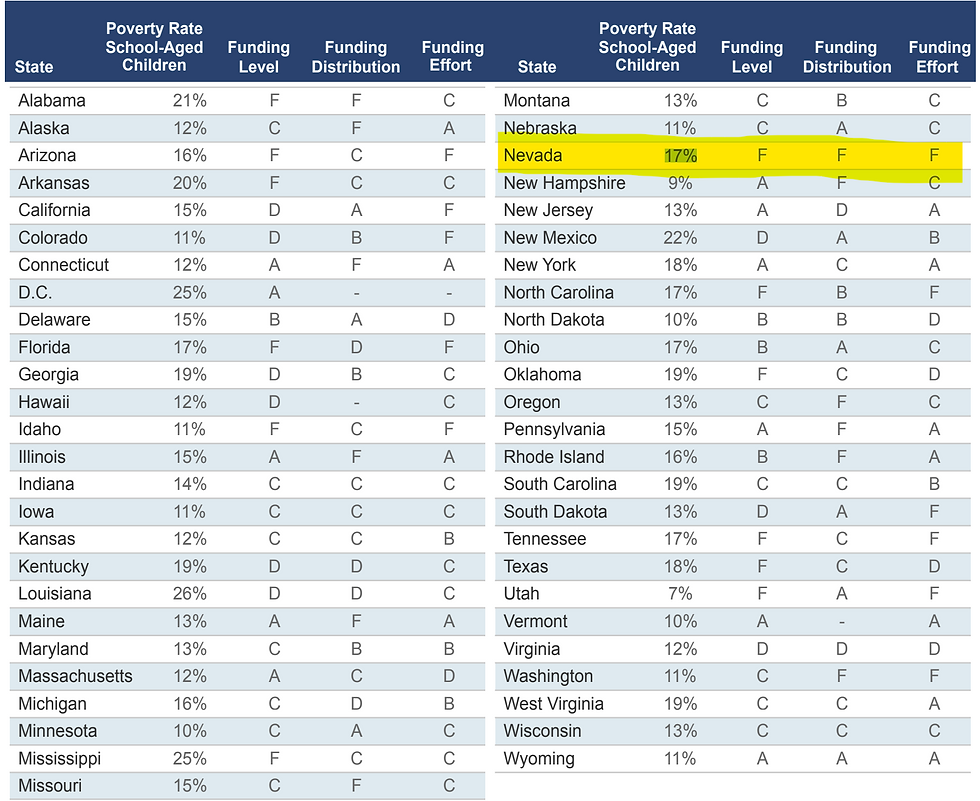
Source: Making the Grade
Enter AI
Infinite Campus has been working in the K-12 space for 30 years - they are not a startup. However, like so many legacy vendors they have added AI/ML to their mix. The state of Nevada, who has used other Infinite Campus products since 2016, now wanted to use machine learning to predict at-risk students, in order to better target them for eligible funding. Note the political choice here. It's not about closing a funding gap or providing better overall supports, but putting more towards those MOST disadvantaged. It’s hard to imagine anyone taking issue with helping disadvantaged kids, but there are also implications in this approach that don’t seem to address the bigger calls to action outlined in the Making the Grade report.
This targeted approach was presumably the motivation behind the turn towards an AI/ML solution. AI/ML was seen to be more accurate or ‘fair’, because it could replace the ‘blunt instrument’ approach of using flawed school lunch enrollments with a more refined set of data inputs - the below noted 75 ‘at risk’ factors, which have been made public.
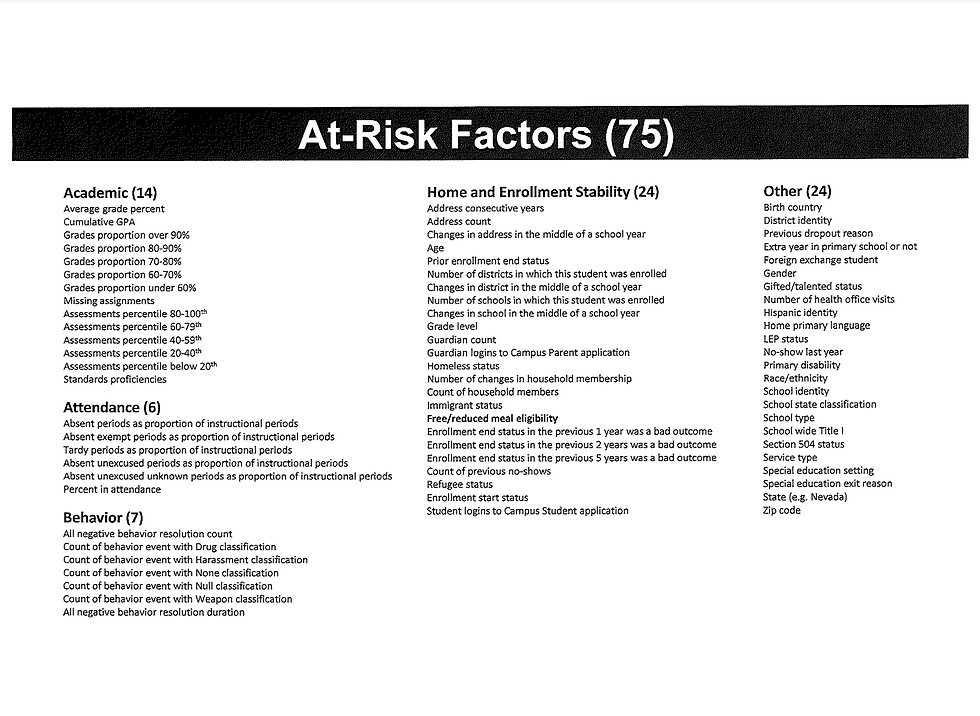
Source: Infinite Campus
The actual weightings of these risk factors in the algorithmic calculation are not public because they are the intellectual property of the vendor. There is some protected category data on this list - notably gender and race, which the company has since said it has removed. This report from New America outlines the problem with the scoring approach:
“The grad scores—which Infinite Campus’ own CEO has likened to a credit score—range from 50 to 150. A cutoff point of 72 determines whether the state will provide additional aid. This new way of defining “at-risk” students reduced the number who were eligible for supplemental state funding from 288,000 in the 2022-23 school year to only 63,000 by the following year. But the needs of the state’s at-risk students didn’t change overnight—only the methods used to identify them.’ - New America
Better or Worse? For whom.
If there is agreement that the goal is to identify at-risk students so that more funding can be directed towards them - which itself is a contested issue - which data is better for achieving that goal and how should it be assessed?
Should low family-income be the sole metric for determining at-risk? Or is it just one of many things to assess and if so, how much weight should it be accorded in the calculation vs other variables?
Is more data better? What is the quality/veracity of these additional data sources?
Does it make sense to still use school lunch enrollment numbers as a proxy for need, in light of the research that suggests it's not a suitable measure for this purpose?
These are social and political questions that AI will not address.
There is also the issue of ‘at-risk of what’? That threshold is graduation. The system is trying to optimize for a prediction of who needs additional assistance to graduate. Is that itself too narrow a framing of ‘at-risk’? What about risks not captured in the data such as depression or risk for self harm? Or the actions of concerned educators who intervene - often on their own time and at their own expense - to make sure students are supported - propping up the data, making it appear better?
It's possible to create a risk scoring system that used a transparent process if it was deemed that having multiple weighted data sources to determine need or 'at-risk' status was indeed a good idea. There seems to be a rush to go from using a single, problematic data point to a ‘black box’ system - incurring all the harms and risks inherent with using predictive AI systems. There are other, less fraught, data analytic techniques that could be applied.
We might also question the limits of risk based thinking as it relates to the domain of education altogether - is this even the right approach? As one teacher expressed in the New York Times piece, she feels 'punished' by the outcomes for doing these extra things to help students. Her under the radar interventions, which prevent the data from becoming bad enough to be deemed risky by the system, are detrimental to receiving funding in this risk assessment approach.
There is so much more to this story when we dig beyond the 'AI gone wrong' headline. That's not to say there are no concerns about using AI - there definitely are! Yet, if we aim to address the issues wrapped up under the banner of Responsible AI, we need to broaden our perspective.
Additional Resources
This piece in Education Week provides additional background on the Nevada story and this assessment by the Markup examines issues of racial bias in systems that predict graduation rates. Infinite Campus has also produced a white paper about their system.
By Katrina Ingram, CEO, Ethically Aligned AI
Ethically Aligned AI is a social enterprise aimed at helping organizations make better choices about designing and deploying technology. Find out more at ethicallyalignedai.com
© 2024 Ethically Aligned AI Inc. All right reserved.