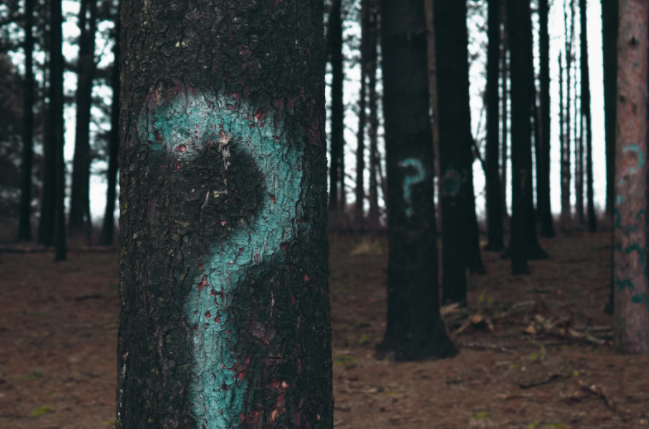
This week I'm getting started on my literature review. I'm trying to craft a list of core values that are part of the DNA of AI systems which might raise ethical concerns. This list is aimed at capturing the values embedded in the AI system itself rather than the AI researchers or designers which will be a separate list. I welcome any feedback on what I’ve noted or what’s missing. I’m also wondering….in considering all of this, should we also ask if AI is the appropriate means to solve the problem at all? When is AI not the right approach? Are we willing to ask that question now? Will it be possible to NOT use AI someday when its super intelligent?
Here’s where I’m at so far:
Efficiency – Cost reduction is a major driver behind the funding of artificial intelligence. AI is designed from a perspective that seeks to find the most efficient process thereby reducing costs. Are there benefits to inefficiency that might be streamlined out of a system? It could be argued that human relationships are inefficient. What does the pursuit of efficiency do to human relationships?
Optimization – AI systems are designed to optimize for X. This implies that optimization is a worthwhile pursuit, that we should want to optimize. This value pairs with efficiency as reducing costs while improving outcomes makes for a compelling rationale for AI. Are there are times when optimization shouldn’t be a goal? Who determines what is optimal (optimal for who)?
Prediction – AI algorithms lend themselves to prediction. They fit historical data to calculate the probability of a future action or occurrence. The same underlying value drives recommendation engines for a movie and screening for a disease. The implication is that we can then use the information to “see the future” and then intervene to drive a desired outcome or prevent an undesirable outcome. Clearly, there are differences in the stakes of the accuracy of the prediction itself when applied to high risk domains like healthcare vs recommending a movie. There are also questions around whether or not intervention is always the right choice.
Scalability – AI systems improve their performance as they scale. This can lead to concentrations of power in companies, governments or other organizations who deliver the most scalable solutions, primarily because they control the resources (data, data scientists, compute). We see this already with GAFA (Google, Amazon, Facebook, Apple). Scale raises questions around monopolies/oligopolies and anti-trust as well as surveillance capitalism. It also touches on the idea of ubiquity – if AI is everywhere, we don’t have the ability to opt out.
Transferability – There are currently a handful of multi-purpose AI algorithms that are applied across domains and a sense from some AI researchers that given enough time, there can be a “one algorithm to rule them all” approach. Is it reasonable to think we can use the same underlying approaches to solve problems in finance, healthcare, the criminal justice system and a long list of other domains? Are we losing any relevant nuances in taking this approach? Are we reframing the problems to fit the solution?
Growth – Related to scalability is the idea that growth is good and that AI supports growth.
Intelligence – The name AI assumes the primacy of intelligence in decision making. AI’s type of logical and probabilistic intelligence is narrow and aligned with its other values (efficiency, optimization, prediction). What about the role of emotion? What about relationships? AI’s form of intelligence is also part of the issue around being explainable. How AI thinks isn’t how we think and in many cases it can’t tell us how it arrives at decisions.
Technological determinism – not specific to AI but aligned with the bigger idea that technology is the driver for socio-economic and political change. The doctrine of progress. It’s also held out as a promise that AI will fix our biggest issues such as climate change. Technology will save us.
Data intensive – Current AI systems are designed to aggregate as much data as possible which serves to better optimize its model. This is a recursive exercise. It’s riddled with numerous ethical concerns around the data (historical bias, lack of representation), how it's gathered, categorized and managed. This is tied to issues around surveillance capitalism noted under scalability.
By Katrina Ingram _______
Sign up for our newsletter to have new blog posts and other updates delivered to you each month!
Ethically Aligned AI is a social enterprise aimed at helping organizations make better choices about designing and deploying technology. Find out more at ethicallyalignedai.com © 2019 Ethically Aligned AI Inc. All right reserved.